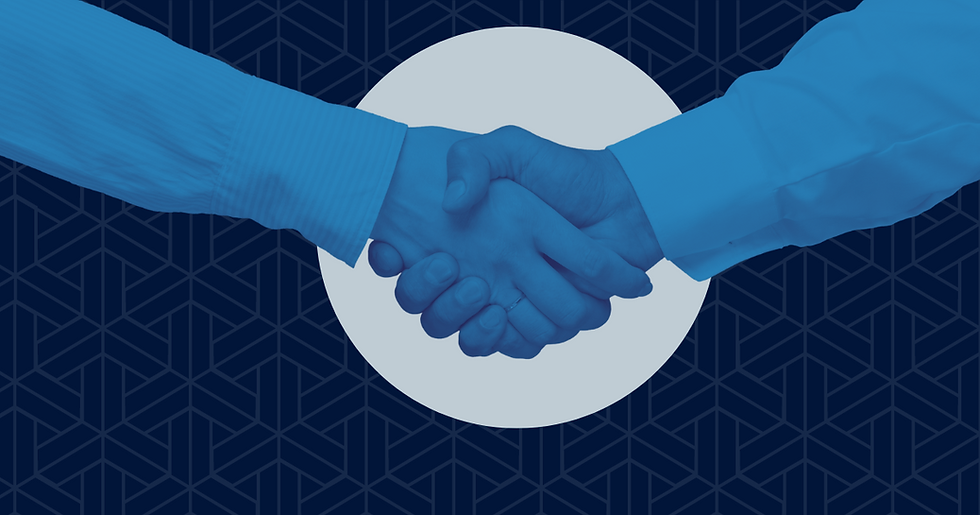
Think of your organisation’s data lakes as large water bodies with lots of H2O molecules (raw data in its native format). As a data owner, you decide if these water molecules get to flow freely and irrigate your organisational decisions or sit stagnantly. Obviously, democratised access to data is what will drive growth and change. But, if data pipelines are not appropriately managed, you get saddled with worries about the quality and security of the data. In turn, it would mean that you hold on tighter to the dataset.
This just won't do.
You need to feel confident about the data and how it will be used -- so that the organisation can benefit from it. In other words, you need to establish trust in data. This is the only way you will feel empowered enough to share data more freely.
Do People ‘Trust your Data’?
Today, companies need to sift through vast amounts of data every day. And with great data volumes comes great responsibilities and remarkable risks. To leverage and protect said data, data owners need to build higher levels of trust in their datasets.
But, what does this ‘trust’ really mean?
You can trust data that is consistent, accurate, complete, timely, traceable, unique and orderly. Once these factors are met, data owners become more comfortable sharing their data. It is also a measure of how confident each department or the analytics team is of the fidelity of datasets. The data should also be protected to satisfy both stakeholder and legal expectations.
Data Trust Challenges to Overcome
Data trust issues are not unusual, nor are they unique to any particular industry. They are both technological and cultural in nature and signify a disconnect between the data requestor and the data owner, and their perception of trust.
According to a study by PwC, data owners’ worries range from data theft and leakage (34%), quality of data (34%), privacy risk from authorized data processing (29%) and data integrity (31%). Thus, they cannot share data if they:
Don't know what data they have - What sort of data is stored in each file? Is there PII (personal) data within the dateset? Is it secured and cleaned?
Don't have absolute control over who can access or change the data
Don't have confidence in the quality and validity of data that will be used to make business decisions.
Data requestors, on the other hand, are equally frustrated for not gaining easy access to data. Unable to prove compliance while processing the data, to data owner; their requests for data access are regularly dismissed. Ultimately, poor access to data means less accurate data for analytics and reduced decision making capabilities.
This is why it's critical to manage data access and assure data understanding, validity and quality. In this way, you create trusted data sources that you don't need to question every time.
5 Ways to Build Trust in Data
The biggest barrier to data ambition lies in convincing data owners to share their precious resources. So, the need of the hour is to capture and process the data in a secure, transparent, governed manner, giving data owners the confidence to share. This ultimately leads to better analytics and compliance.
Here are some of the best tactics that can help build the health of your data pipelines:
Get data cleaned and validated: Analytics teams wouldn’t be able to trust in the data to make business decisions if it is fraught with duplications, lack of consistency and timeliness, etc. Basically, if you ingest garbage data, you will receive garbage business insights. Therefore, you need to strive for accurate, consistent, complete and reliable data to build user trust.
Add metadata and business logic: Metadata and business logic add context to each piece of data so that data owners can precisely map the contents of pipelines. By significantly improving the business and technical understanding of your data, it enhances the data searchability and sensitive data discovery.
Secure sensitive data: Often, you need to mask and hide PII data to avoid non-compliance around GDPR. Dynamic data masking solution by Bluemetrix allows you to consistently anonymise, de-tokenise & de-identify such data before disclosure.
Monitor and track data: To trust your data, you must have the ability to prove that trust. For instance, you must have the ability to check where a record has been and the journey it has taken within your systems. You should also be able to tell who has accessed it throughout that journey. Bluemetrix’s schema evolution and versioning system scalably monitor and track what happens to data. This ensures that data consistency is guaranteed between data sources and destinations.
Gain complete visibility and control over the process: Chaos would reign if data owners have no control over or view of who can access or change the data. Hence, data owners should strive to ensure a detailed audit trail reporting to know how and when the data has evolved. Also, by applying tags to different parts of the pipeline, you can devise unique data access policies.
By optimising every step of the data value chain, you turn questionable data into valuable data. Once you secure stakeholder’s trust in data, you can harness it while respecting customer privacy and honouring regulations.
With data lakes ingesting thousands of different pipelines from multiple departments, manually undertaking such data optimisation can be exhausting. The best workaround would be to invest time to identify an automated data governance solution that enables your stakeholders to establish trust in data.
Comments